AI’s impact on the supply chain is often limited to demand forecasting, which it can significantly improve. However, the supply chain challenge is much larger than that. The movement of physical products, components, and raw materials across multiple facilities and geographies creates numerous intrinsic and extrinsic obstacles that AI can help address.
The increasing availability of low-code AI tools is enabling more organizations to leverage artificial intelligence in their operations. With sophisticated tools that can handle end-to-end machine learning operations and foundational models for language, image, and vision, organizations can rapidly extract value from their data. In addition to this, self-supervised learning techniques are making it possible to train models with less labeled data, which is a major breakthrough for organizations with limited access to high-quality training data. Furthermore, recent advancements in large language models (LLMs) are improving the ability to process vast amounts of data and facilitate meaningful interactions with stakeholders in the ecosystem. The diagram below provides an overview of AI use cases in the supply chain, and generative AI holds tremendous potential for harnessing the data and insights generated by these use cases.
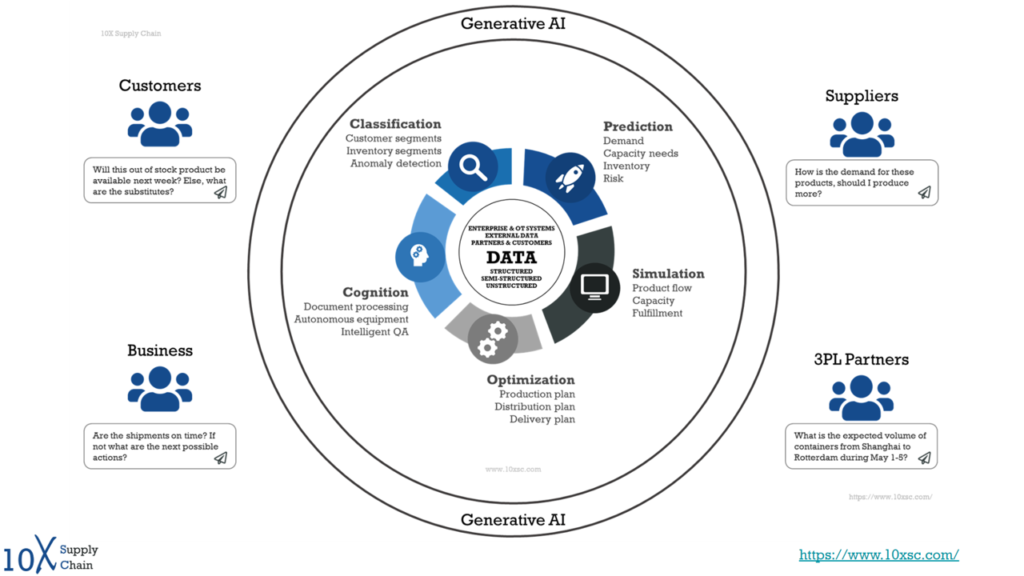